On-Premise AI Servers: A high growth & underserved market
I recently adviced a European IT service provider to explore offering on-premise AI server solutions. I believe it is a high growth and underserved market.
On-premise AI servers involve installing AI hardware within a company, setting up models, fine-tuning them with proprietary data, and itegrating them with existing systems.
Why should a company do all this and not just use chatGPT or something similar? I analyze this below:
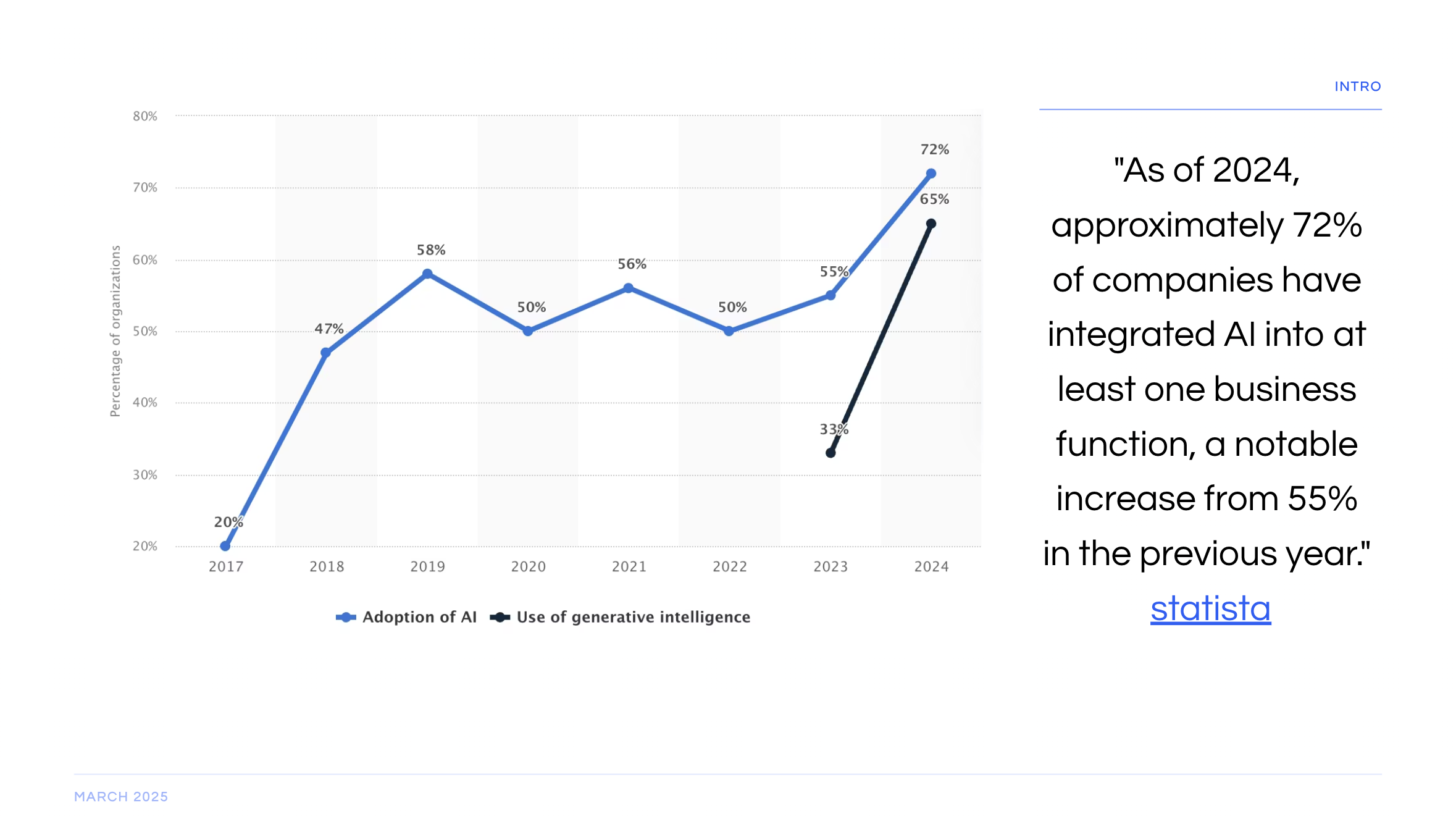
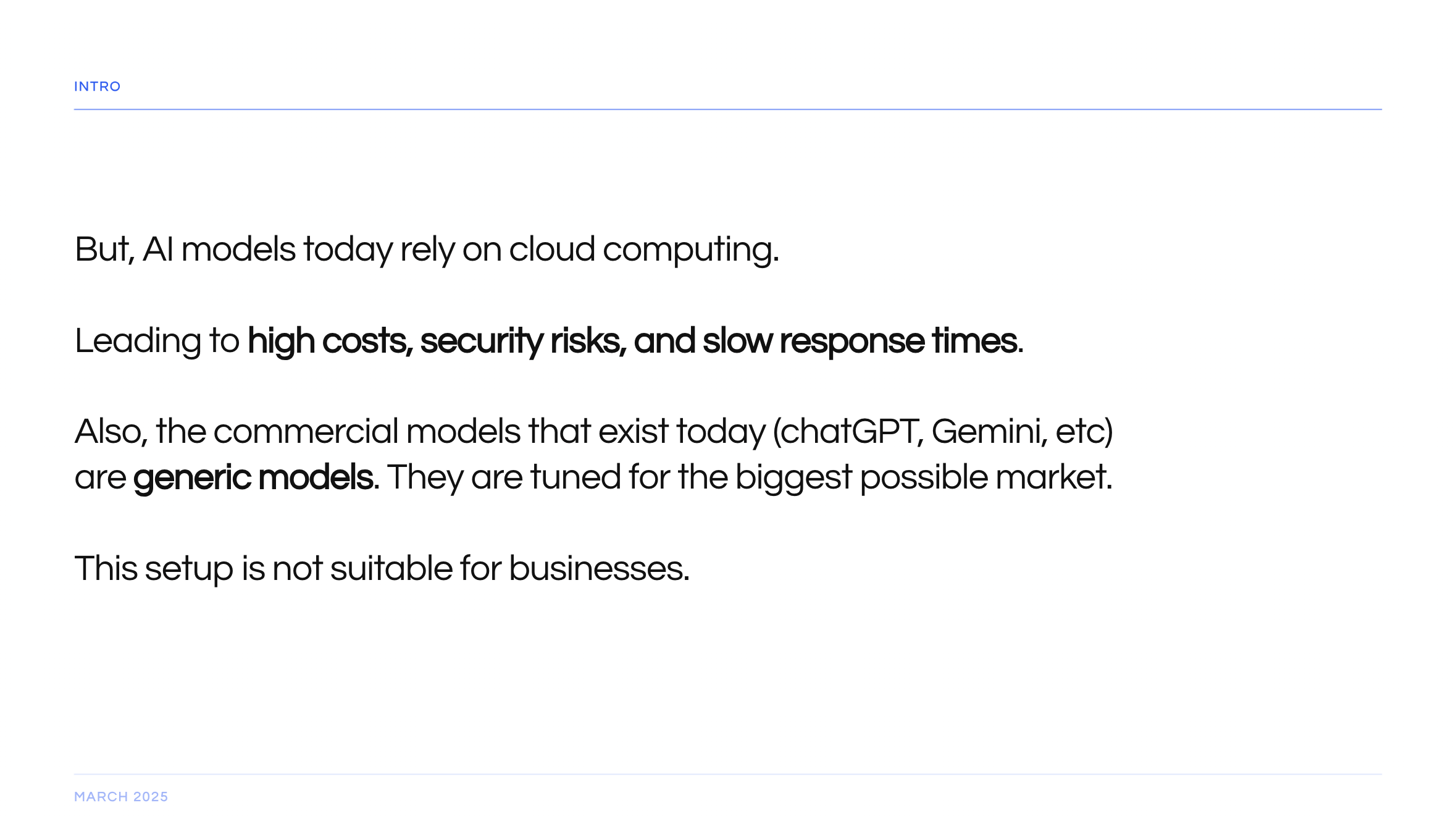
Data privacy is one of the biggest reasons why on-prem AI will be big. The argument is even bigger in Europe since there is high regulation on data privacy (EU Data Act). They are also pushing AI infrastructure (see EU launches InvestAI initiative to mobilise €200 billion of investment in artificial intelligence).
First of all, AI is an umbrella term. The current hype has been about Generative AI where the AI is generating text, images, and videos. OpenAI, brought the heat with a subcategory of Generative AI: Large Language Models. Their biggest product is chatGPT.
Of course there are other uses for AI, not just Generative AI. There's fraud detection in banks, trading algorithms in trading, quality control in assembly lines.
Back to chatGPT. Whenever someone asks a question, uploads a document, or shares information in any way, that data is automatically in the system. For example, chatGPT can be used as an advisor. If we utilize it to ask questions for [Our Company] and input all financial statements and client's data, all this data is now used to train the system. So if someone is investigating [Our Company Name] and starts asking questions, there is a non-zero chance that our data are shown to that individual. It is a -very- long shot, but possible. Meaning, it is a huge privacy issue. And Europe is cracking down on privacy issues. "Under GDPR in Europe, personal data must be protected and often kept within specified geographic boundaries. Third-party Cloud AI (especially from non-EU providers) carries legal risk, since transferring EU citizen data to foreign cloud servers could breach GDPR."
Also, third-party Cloud AI means sensitive data is stored on third-party servers, raising concerns about breaches and unauthorized access. Of course, the same argument could be said about third-party Cloud Storage solutions. Why is it more dangerous to share sensitive data on third-party Cloud AI than third-party Cloud Storage solutions?
Because:
- AI services involve continuous data processing -meaning more frequent data transfers, copies, and transformations, significantly increasing exposure risks.
- Third-party Cloud AI may process sensitive data externally in ways less transparent than simple cloud storage, making it harder to audit and control exactly how data is accessed or used, and raising compliance complexities.
- Unlike third-party Cloud Storage, third-party AI operations might require integrating sensitive data deeply into proprietary AI models, increasing the risk of vendor lock-in and regulatory complications if companies decide to move or withdraw later.
It is highly probable that there are going to be big fines on European companies using third-party Cloud AI services. Maybe, that is what will trigger the on-prem AI solution trend. Until then, it's first mover's advantage.
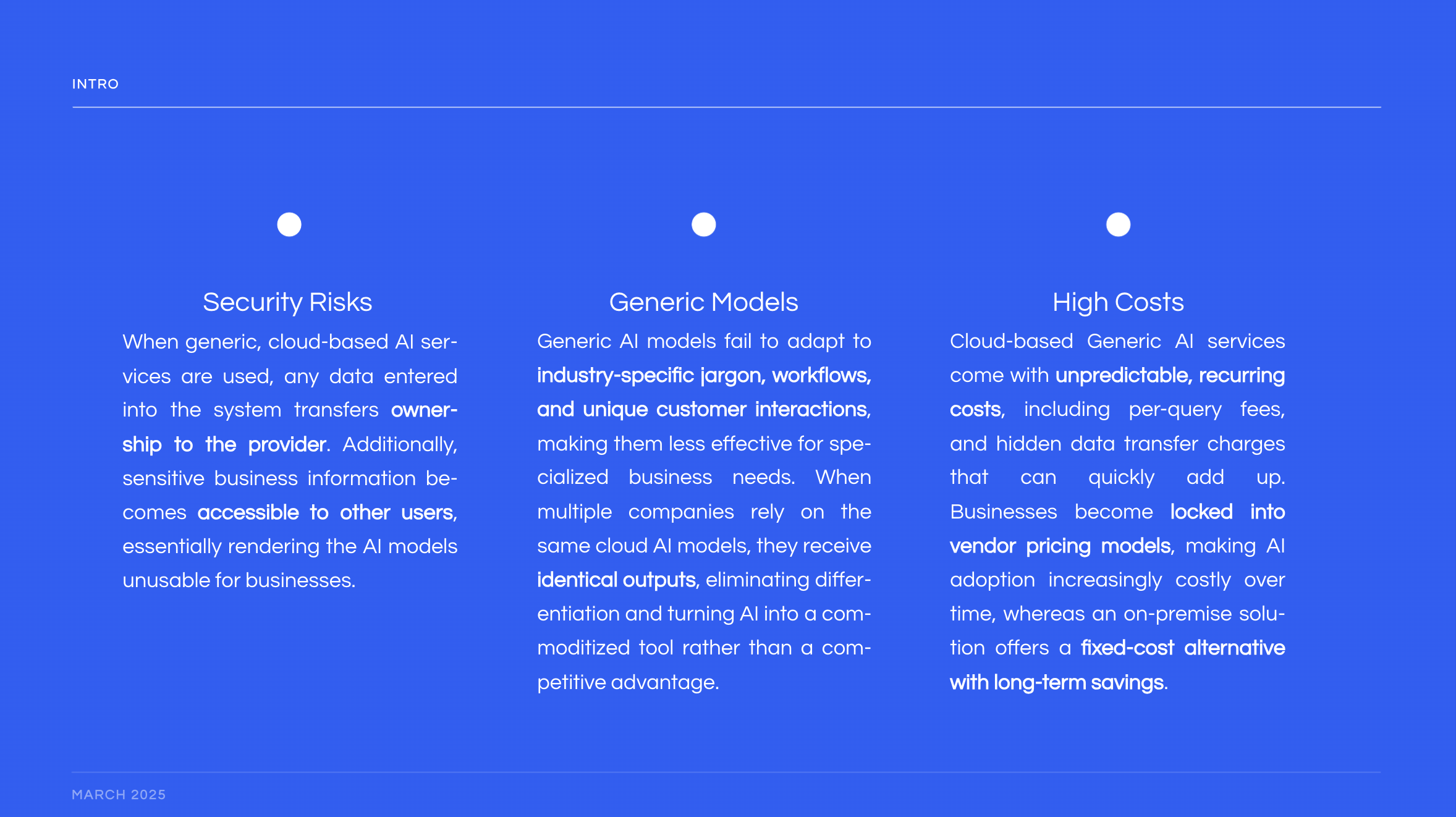
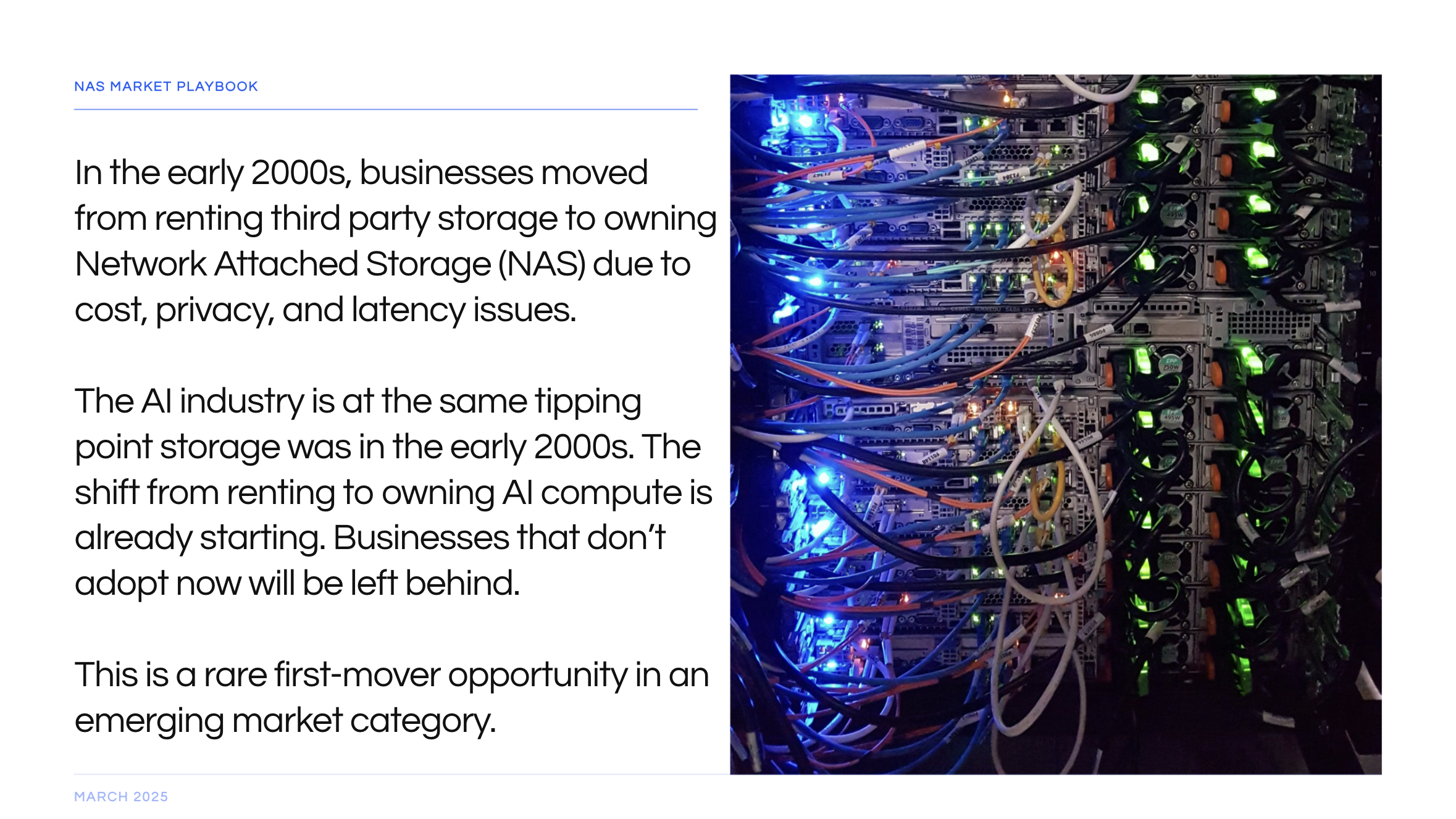
Cost: The truth is, on-prem AI involves higher up-front hardware investment but offers more predictable ongoing costs.
It is hard to accurately calculate the cost of third-party AI services because of their business model. They charge per 'token' which is aproximately 4 characters. There are also different charges based on the tokens we input (our query) and the tokens the AI generates (the response).
But Enterprises shift to on-premises AI to control costs. Enterprises have used these systems at scale. So, they have the data to prove that the upfront investment in on-prem AI services makes financial sense.
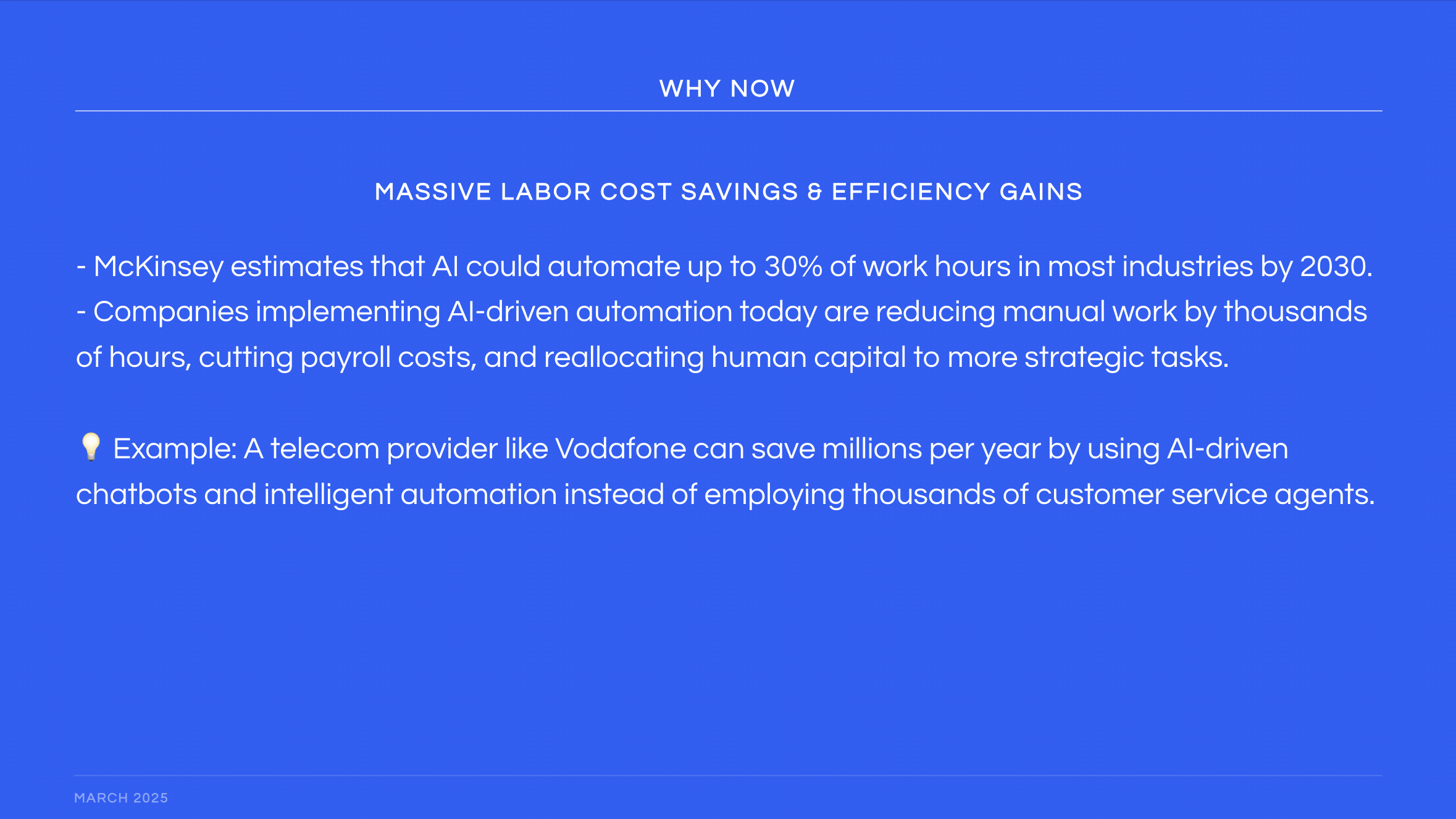
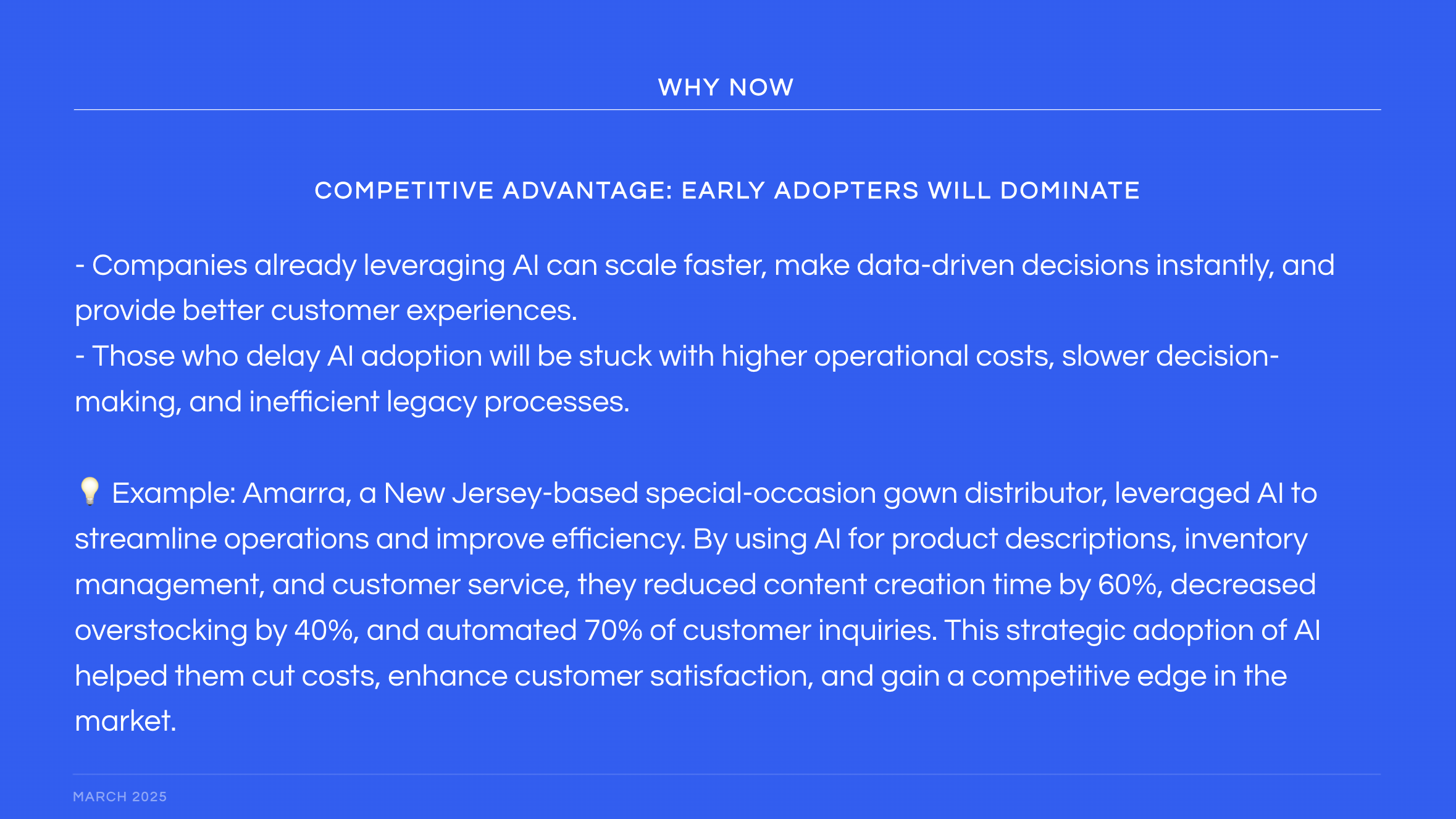
The third argument is that models like chatGPT are generic models. This is not optimal for business use.
First of all, a big misunderstanding about Large Language Models (like chatGPT) is that they are perceived as knowledge bases. They are not. They are logic machines. They are trained on vast amounts of text data (they've scraped most of the internet) and used the data to find patterns. In a very simple example, if you ask: "It is cloudy outside and will probably rain, I should get an..." it will respond "umbrella". That is because this is the most common pattern. Of course, they can provide information they have used to be trained on. For example, you can ask for Shakespeare's Romeo & Juliet and it will generate the play for you to read. You can also ask it to generate the play in the style of Stephen King and it will do so. But if you ask it to generate it in your style, it does not have enough data to do so. The solution to that would be to provide it with as much as of your writings as possible.
As a bigger example, let's say Vodafone is to create a customer service chat bot on their website. This will allow them to cut costs on human agents. But it does not make sense for them to upload all their internal and public documents and their customer's data to train chatGPT. What they have to do is to train their own model so they have consistent, on brand, and factually correct responses. They could do so with third-party AI solution providers but then the privacy and cost issues come along. What they should do is use an on-prem AI solution and train their own model.
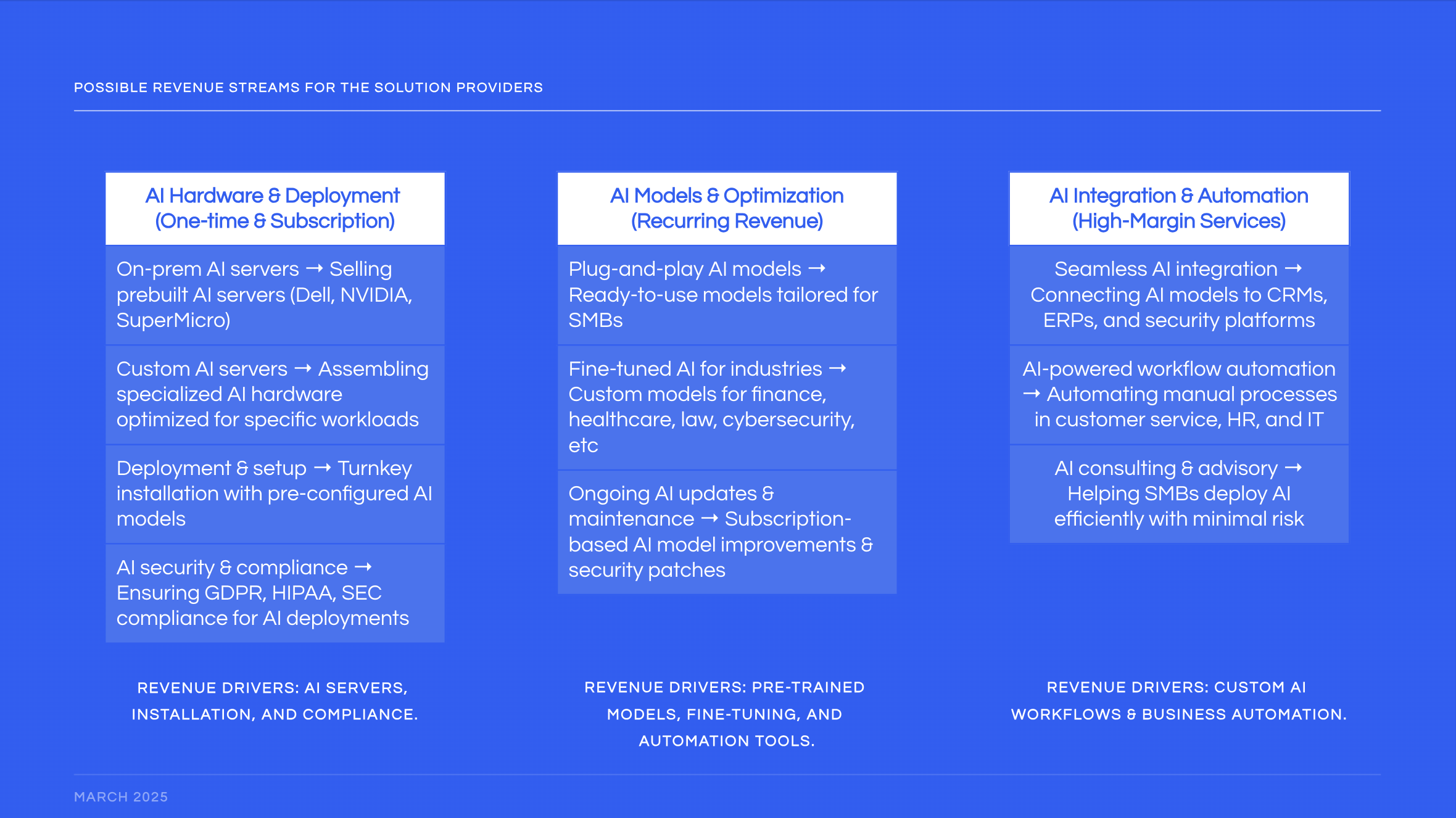
Now, who is buying? Applications for this are in every business. It would make sense, for a solution provider, to begin with higher revenue businesses (banks, finance, retail) than lower ones, like education.
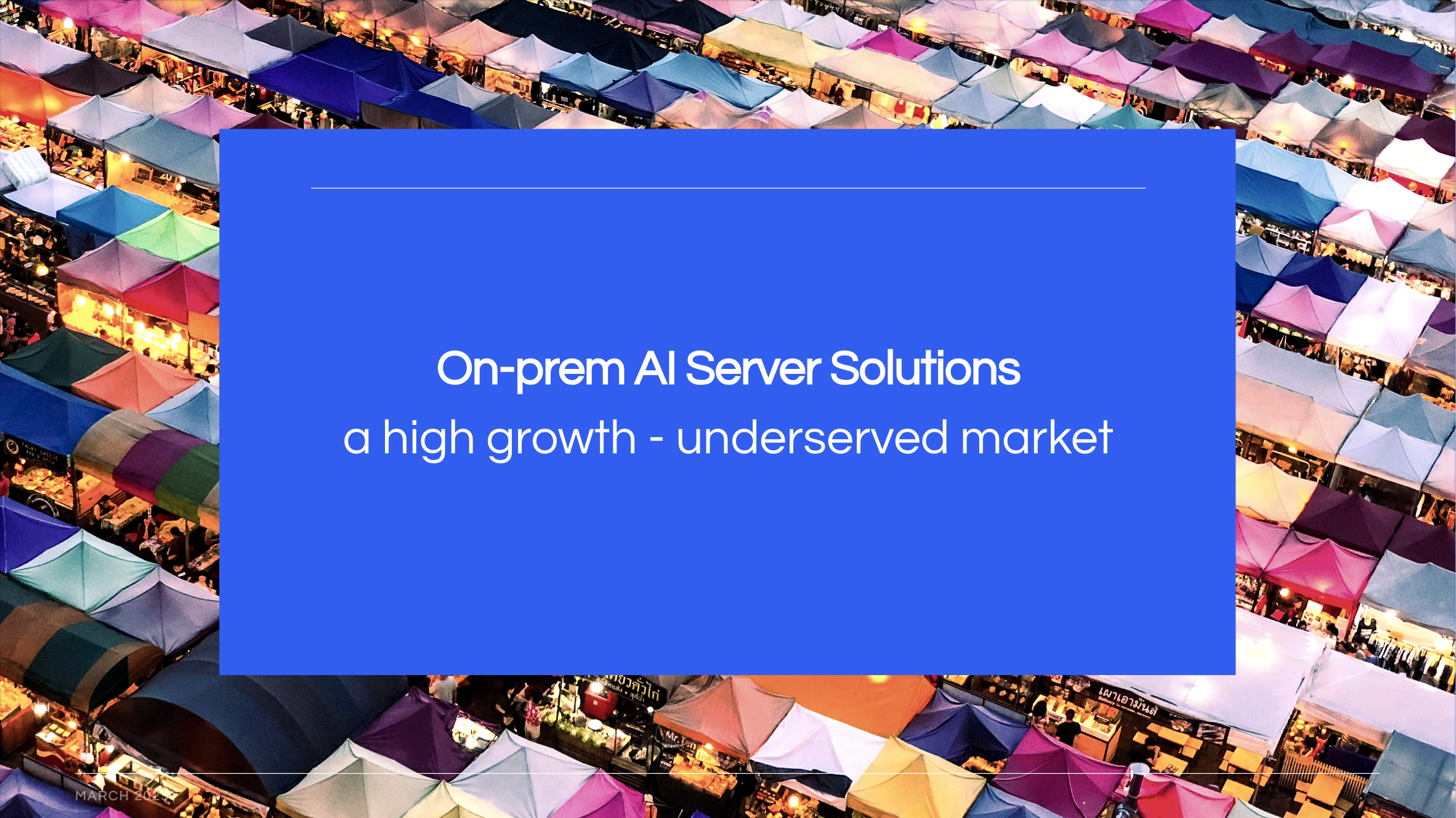